A while back I went to one of these specifically for writing an F31 NRSA fellowship application. Ended up getting one the first try. I thought the workshop was helpful. This looks a little more general, but may give you some ideas about getting external funding that isn't necessarily from NIH.
Grant & Fellowship Workshop for Graduate Students
March 4, 2010
5:30-7:30pm
Buttrick Hall
Registration link
Friday, February 26, 2010
Seminar: Spectral Anonymization Data
Thomas Lasko, MD, PhD, from Google Inc., Mountain View CA, will be giving a seminar next week entitled:
"Spectral Anonymization Data"
March 3, 2010
12:00 - 1:00 p.m.
214 Light Hall
Abstract:
The great challenge of data anonymization is to condition a dataset for public release such that it remains scientifically useful, but it does not disclose sensitive information about the individuals it describes. The challenge arises every time we consider releasing a clinical dataset, and with genomic data the problem is orders of magnitude more difficult. It is not sufficient to simply remove directly identifying information, because the remaining clinical data may be enough to infer an identity or otherwise learn sensitive information about an individual, especially if the attacker has access to auxiliary information.
Data anonymization has been an area of active research for several decades, yet almost every aspect of it remains an open question: How do we measure the risk of disclosure, and what amount of risk is acceptable? What is the optimal method of perturbing the data to achieve this protection? How do we measure the impact of the perturbation on scientific analysis, and what is an acceptable impact? Dozens of anonymization methods have been proposed over the years, but none has been successful at simultaneously providing perfect privacy protection and allowing perfectly accurate scientific analysis. One respected researcher opined in print that for data involving more than a few dimensions, the anonymization problem appears impossible.
In this talk, I make the claim that the problem is not impossible, but historically we have imposed unnecessary and conflicting constraints on it. By relaxing these constraints and working under strong and defensible definitions of privacy and utility, we can simultaneously achieve both perfect privacy and perfect utility, even in high dimension. I demonstrate how the principle of Spectral Anonymization relaxes some of these unnecessary constraints, and I present a concrete algorithm that achieves practically perfect privacy and utility using spectral kernel methods of machine learning.
"Spectral Anonymization Data"
March 3, 2010
12:00 - 1:00 p.m.
214 Light Hall
Abstract:
The great challenge of data anonymization is to condition a dataset for public release such that it remains scientifically useful, but it does not disclose sensitive information about the individuals it describes. The challenge arises every time we consider releasing a clinical dataset, and with genomic data the problem is orders of magnitude more difficult. It is not sufficient to simply remove directly identifying information, because the remaining clinical data may be enough to infer an identity or otherwise learn sensitive information about an individual, especially if the attacker has access to auxiliary information.
Data anonymization has been an area of active research for several decades, yet almost every aspect of it remains an open question: How do we measure the risk of disclosure, and what amount of risk is acceptable? What is the optimal method of perturbing the data to achieve this protection? How do we measure the impact of the perturbation on scientific analysis, and what is an acceptable impact? Dozens of anonymization methods have been proposed over the years, but none has been successful at simultaneously providing perfect privacy protection and allowing perfectly accurate scientific analysis. One respected researcher opined in print that for data involving more than a few dimensions, the anonymization problem appears impossible.
In this talk, I make the claim that the problem is not impossible, but historically we have imposed unnecessary and conflicting constraints on it. By relaxing these constraints and working under strong and defensible definitions of privacy and utility, we can simultaneously achieve both perfect privacy and perfect utility, even in high dimension. I demonstrate how the principle of Spectral Anonymization relaxes some of these unnecessary constraints, and I present a concrete algorithm that achieves practically perfect privacy and utility using spectral kernel methods of machine learning.
Tags:
Announcements
Wednesday, February 24, 2010
Student Invited Seminar: Developing Effective Science Advocates – Making the case for increased funding for biomedical research
The Pharmacology department's student invited seminar is coming up next week. Last time the students invited an excellent speaker from Washington to talk about AAAS fellowships.
This year's student invited speaker is James S. Bernstein, Director, Government and Public Affairs for the American Society for Pharmacology and Experimental Therapeutics.
"Developing Effective Science Advocates – Making the case for increased funding for biomedical research"
Monday, March 1, 2010
2:00 pm in 206 PRB
This year's student invited speaker is James S. Bernstein, Director, Government and Public Affairs for the American Society for Pharmacology and Experimental Therapeutics.
"Developing Effective Science Advocates – Making the case for increased funding for biomedical research"
Monday, March 1, 2010
2:00 pm in 206 PRB
Tags:
Announcements,
Policy
Tuesday, February 23, 2010
Happy Birthday GGD! The 10 Most Popular Posts Since GGD's Launch
The first post on Getting Genetics Done was one year ago today. To celebrate, here are the top 10 most viewed posts since GGD launched last year. Incidentally, nine of the ten are tutorials on how to do something in R. Thanks to all the readers and all the commenters for sharing your thoughts!
ggplot2 Tutorial: Scatterplots in a Series of Small Multiples
GWAS Manhattan plots and QQ plots using ggplot2 in R
Hierarchical Clustering in R (by Will Bush)
Comparison of plots using Stata, R base, R lattice, and R ggplot2: Histograms
Merge data from different files using R
QQ plots of p-values in R using ggplot2
PDF tutorial from R course (Introduction to R)
Visualizing sample relatedness in a GWAS using PLINK and R
Split, apply, and combine in R using PLYR
Linux Command Line Cheat Sheet
ggplot2 Tutorial: Scatterplots in a Series of Small Multiples
GWAS Manhattan plots and QQ plots using ggplot2 in R
Hierarchical Clustering in R (by Will Bush)
Comparison of plots using Stata, R base, R lattice, and R ggplot2: Histograms
Merge data from different files using R
QQ plots of p-values in R using ggplot2
PDF tutorial from R course (Introduction to R)
Visualizing sample relatedness in a GWAS using PLINK and R
Split, apply, and combine in R using PLYR
Linux Command Line Cheat Sheet
Tags:
ggplot2,
GWAS,
Linux,
PLINK,
R,
Stata,
Statistics,
Tutorials,
Visualization
Monday, February 22, 2010
JMP Genomics Workshop
Doug Robinson (JMP Academic Division, SAS Institute) is giving a presentation of the JMP Genomics software from SAS illustrating data analysis for genetics, expression, and copy number variation studies. Agenda includes: CNV analysis, merging CNV data sets of continuous traits, and QC, analysis, and downstream applications of expression studies.
Tuesday Feb 23, 11 am
512 Light Hall
Tuesday Feb 23, 11 am
512 Light Hall
Tags:
Announcements,
Software,
Statistics
Wednesday, February 17, 2010
"Synthetic associations" - an alternative point of view
If you read the previously mentioned "synthetic associations" paper in PLoS Bio by Dickson et al. in David Goldstein's group, be sure to read this brief commentary written by Pat Sullivan at UNC offering a different point of view. The original paper created the liveliest discussion we've had in journal club since we talked about the ISC en masse paper, and Sullivan's commentary very nicely mirrors some of our own thoughts on the paper that were brought up at journal club last week.
Patrick Sullivan: “Synthetic” Ado About Nothing?
Patrick Sullivan: “Synthetic” Ado About Nothing?
Tags:
GWAS,
Journal club,
Recommended Reading
Tuesday, February 16, 2010
ROADTRIPS: Case-Control Association Testing with Partially or Completely Unknown Population and Pedigree Structure
A tip of the hat to @JVJAI for pointing out this interesting looking paper in AJHG.
ROADTRIPS: Case-Control Association Testing with Partially or Completely Unknown Population and Pedigree Structure
Timothy Thornton and Mary Sara McPeek
Department of Biostatistics, University of Washington.
Abstract: Genome-wide association studies are routinely conducted to identify genetic variants that influence complex disorders. It is well known that failure to properly account for population or pedigree structure can lead to spurious association as well as reduced power. We propose a method, ROADTRIPS, for case-control association testing in samples with partially or completely unknown population and pedigree structure. ROADTRIPS uses a covariance matrix estimated from genome-screen data to correct for unknown population and pedigree structure while maintaining high power by taking advantage of known pedigree information when it is available. ROADTRIPS can incorporate data on arbitrary combinations of related and unrelated individuals and is computationally feasible for the analysis of genetic studies with millions of markers. In simulations with related individuals and population structure, including admixture, we demonstrate that ROADTRIPS provides a substantial improvement over existing methods in terms of power and type 1 error. The ROADTRIPS method can be used across a variety of study designs, ranging from studies that have a combination of unrelated individuals and small pedigrees to studies of isolated founder populations with partially known or completely unknown pedigrees. We apply the method to analyze two data sets: a study of rheumatoid arthritis in small UK pedigrees, from Genetic Analysis Workshop 15, and data from the Collaborative Study of the Genetics of Alcoholism on alcohol dependence in a sample of moderate-size pedigrees of European descent, from Genetic Analysis Workshop 14. We detect genome-wide significant association, after Bonferroni correction, in both studies.
ROADTRIPS: Case-Control Association Testing with Partially or Completely Unknown Population and Pedigree Structure
Timothy Thornton and Mary Sara McPeek
Department of Biostatistics, University of Washington.
Abstract: Genome-wide association studies are routinely conducted to identify genetic variants that influence complex disorders. It is well known that failure to properly account for population or pedigree structure can lead to spurious association as well as reduced power. We propose a method, ROADTRIPS, for case-control association testing in samples with partially or completely unknown population and pedigree structure. ROADTRIPS uses a covariance matrix estimated from genome-screen data to correct for unknown population and pedigree structure while maintaining high power by taking advantage of known pedigree information when it is available. ROADTRIPS can incorporate data on arbitrary combinations of related and unrelated individuals and is computationally feasible for the analysis of genetic studies with millions of markers. In simulations with related individuals and population structure, including admixture, we demonstrate that ROADTRIPS provides a substantial improvement over existing methods in terms of power and type 1 error. The ROADTRIPS method can be used across a variety of study designs, ranging from studies that have a combination of unrelated individuals and small pedigrees to studies of isolated founder populations with partially known or completely unknown pedigrees. We apply the method to analyze two data sets: a study of rheumatoid arthritis in small UK pedigrees, from Genetic Analysis Workshop 15, and data from the Collaborative Study of the Genetics of Alcoholism on alcohol dependence in a sample of moderate-size pedigrees of European descent, from Genetic Analysis Workshop 14. We detect genome-wide significant association, after Bonferroni correction, in both studies.
Tags:
GWAS,
Recommended Reading,
Statistics
Monday, February 15, 2010
Keep your lab notebook in a private blog
In my previous post about Q10 a commenter suggested a software called "The Journal" by davidRM for productively keeping track of experiments, datasets, projects, etc. I've never tried this software before, but about a year ago I ditched my pen and paper lab notebook for an electronic lab notebook in the form of a blog using Blogger, the same platform I use to write Getting Genetics Done.
The idea of using a blogging platform for your lab notebook is pretty simple and the advantages are numerous. All your entries are automatically dated appear chronologically. You can view your notebook or make new entries from anywhere in the world. You can copy and paste useful code snippets, upload images and documents, and take advantages of tags and search features present with most blogging platforms. I keep my lab notebook private - search engines can't index it, and I have to give someone permission to view before they can see it. Once you've allowed someone to view your blog/notebook, you can also allow them to comment on posts. This is a great way for my mentor to keep track of result and make suggestions. And I can't count how many times I've gone back to an older notebook entry to view the code that I used to do something quickly in R or PLINK but didn't bother to save anywhere else.
Of course Blogger isn't the only platform that can do this, although it's free and one of the easiest to get set up, especially if you already have a Google account. Wordpress is very similar, and has tons of themes. You can find lots of comparisons between the two online. If you have your own web host, you can install the open-source version of Wordpress on your own host, for added security and access control (see this explanation of the differences between Wordpress.com and Wordpress.org).
Another note-taking platform I've been using recently is Evernote. Lifehacker blog has a great overview of Evernote's features. It runs as a desktop application, and it syncs across all your computers, and online in a web interface also. The free account lets you sync 40MB a month, which is roughly the equivalent of 20,000 typed notes. This quota resets every month, and you start fresh at 40MB. You can also attach PDFs to a note, and link notes to URLs. Every note is full-text searchable.
And then of course there's the non-free option: Microsoft OneNote. Although it will set you back a few bucks, it integrates very nicely with many features on your Windows machine. I've never used OneNote.
The idea of using a blogging platform for your lab notebook is pretty simple and the advantages are numerous. All your entries are automatically dated appear chronologically. You can view your notebook or make new entries from anywhere in the world. You can copy and paste useful code snippets, upload images and documents, and take advantages of tags and search features present with most blogging platforms. I keep my lab notebook private - search engines can't index it, and I have to give someone permission to view before they can see it. Once you've allowed someone to view your blog/notebook, you can also allow them to comment on posts. This is a great way for my mentor to keep track of result and make suggestions. And I can't count how many times I've gone back to an older notebook entry to view the code that I used to do something quickly in R or PLINK but didn't bother to save anywhere else.
Of course Blogger isn't the only platform that can do this, although it's free and one of the easiest to get set up, especially if you already have a Google account. Wordpress is very similar, and has tons of themes. You can find lots of comparisons between the two online. If you have your own web host, you can install the open-source version of Wordpress on your own host, for added security and access control (see this explanation of the differences between Wordpress.com and Wordpress.org).
Another note-taking platform I've been using recently is Evernote. Lifehacker blog has a great overview of Evernote's features. It runs as a desktop application, and it syncs across all your computers, and online in a web interface also. The free account lets you sync 40MB a month, which is roughly the equivalent of 20,000 typed notes. This quota resets every month, and you start fresh at 40MB. You can also attach PDFs to a note, and link notes to URLs. Every note is full-text searchable.
And then of course there's the non-free option: Microsoft OneNote. Although it will set you back a few bucks, it integrates very nicely with many features on your Windows machine. I've never used OneNote.
Tags:
Productivity,
Software,
Web Apps,
Writing
Friday, February 12, 2010
Seminar: Statistical Methods for DNA Resequencing Analysis in Disease-Gene Studies
Department of Biostatistics Seminar/Workshop Series:
Statistical Methods for DNA Resequencing Analysis in Disease-Gene Studies
Wenyi Wang, Ph.D., Faculty Candidate
Stanford Genome Technology Center, UC Berkeley Statistics
2:00-3:00pm
Monday, February 15, 2010
MRB III Room 1220
Intended Audience: Persons interested in applied statistics, statistical theory, epidemiology, health services research, clinical trials methodology, statistical computing, statistical graphics, R users or potential users
Nuclear genes encode most mitochondrial proteins and their mutations cause diverse and debilitating clinical disorders. To date, 1,200 genes have been recorded to be associated with mitochondrial diseases. Identifying DNA variants in these genes in individuals affected by mitochondrial diseases remains a major challenge, because many diseases are thought to be associated with rare variants (minor allele frequency <1%). Medical resequencing arrays enable cost-efficient and high-throughput sequencing of candidate genes. For diploid genomes, available base-calling tools only achieve high accuracy by calling a portion of all nucleotide positions.
Distinct from whole-genome SNP data, the array-based resequencing data present a very low frequency of biological signals (genetic variations from a reference sequence), which motivated us to develop a new statistical method, SRMA (Sequence Robust Multi-array Analysis). Our challenge was to fully detect sequence variations with minimal false discoveries, when sequencing errors occur at high frequencies. We extended the multi-level mixture models, previously deployed for SNP arrays, to accurately call single heterozygous samples at rare variant positions. We demonstrate our methods in a resequencing study of 39 candidate genes among healthy individuals and patients with mitochondrial diseases.
Statistical Methods for DNA Resequencing Analysis in Disease-Gene Studies
Wenyi Wang, Ph.D., Faculty Candidate
Stanford Genome Technology Center, UC Berkeley Statistics
2:00-3:00pm
Monday, February 15, 2010
MRB III Room 1220
Intended Audience: Persons interested in applied statistics, statistical theory, epidemiology, health services research, clinical trials methodology, statistical computing, statistical graphics, R users or potential users
Nuclear genes encode most mitochondrial proteins and their mutations cause diverse and debilitating clinical disorders. To date, 1,200 genes have been recorded to be associated with mitochondrial diseases. Identifying DNA variants in these genes in individuals affected by mitochondrial diseases remains a major challenge, because many diseases are thought to be associated with rare variants (minor allele frequency <1%). Medical resequencing arrays enable cost-efficient and high-throughput sequencing of candidate genes. For diploid genomes, available base-calling tools only achieve high accuracy by calling a portion of all nucleotide positions.
Distinct from whole-genome SNP data, the array-based resequencing data present a very low frequency of biological signals (genetic variations from a reference sequence), which motivated us to develop a new statistical method, SRMA (Sequence Robust Multi-array Analysis). Our challenge was to fully detect sequence variations with minimal false discoveries, when sequencing errors occur at high frequencies. We extended the multi-level mixture models, previously deployed for SNP arrays, to accurately call single heterozygous samples at rare variant positions. We demonstrate our methods in a resequencing study of 39 candidate genes among healthy individuals and patients with mitochondrial diseases.
Tags:
Announcements,
R,
Sequencing,
Statistics
Thursday, February 11, 2010
Using MySQL to Store and Organize Results from Genetic Analyses
This is the first in a series of posts on how to use MySQL with genetic data analysis. MySQL is a very popular, freely available database management system that is easily installed on desktop computers (or Linux servers). The "SQL" in MySQL stands for Structured Query Language, which is by my humble estimation the most standardized way to store and access information in the known universe. SQL is an easy language to learn, and is fundamentally based on set operations such as unions and intersects.
Over the course of this tutorial, I'll illustrate how to use MySQL to load, store, access, and sort SNP statistics computed by PLINK. To begin, you will need to download and install MySQL on your local machine, and you'll also need to install the MySQL GUI tools.
You can think of MySQL as a massive spreadsheet application like Excel - The basic unit within the database is a table, consisting of rows (sometimes called tuples) and columns (generally called fields). A collection of tables is called a schema (the plural form is schemata) - schemata are typically used for organizational purposes.
After installing MySQL Server and the MySQL GUI Tools, start the MySQL Query Browser. The Query Browser will prompt you for connection information - this information will depend on where you installed the MySQL Server application. Assuming that you installed the Server on your current computer, you would enter "localhost" as the host. Also, when installing MySQL Server, you were required to enter a password for the "root" account (which is the primary administrative account). Enter "root" as the username and the password you specified during setup.
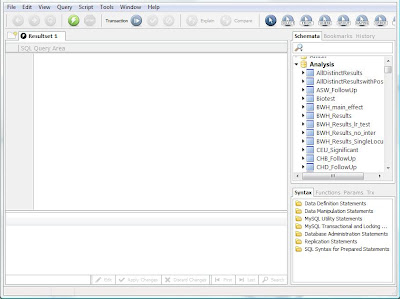
Now you should see the MySQL Query Browser, which has three main panes: the SQL query area, the schemata list, and the results pane. They schemata list is useful for browsing what the database contains, and allows you to see the field names and tables for each schema. The query editor allows you to enter and execute an SQL statement, and the results of that statement are returned in the results pane, which is essentially a read-only spreadsheet.
Lets start by creating a new schema to hold the tables we will make in this tutorial. In the Query Browser, type:
Now let's create a table in the Sandbox schema. If you haven't already, double-click the Sandbox schema in the schemata browser to make it the active schema, then execute the following statement:
This statement created a table called Tutorial in the Sandbox schema that has 3 fields: a character-based field with a max of 30 characters called "name", a character-based field with a max of 150 characters called "address", and an integer-based field called "zipcode".
Now let's load some data into this table. Most genetic analysis applications will output a text file with analysis results -- with this in mind, we'll create a text file containing several names, addresses, and zip codes to load into our new table:
The keyword LOCAL tells the MySQL browser to use files on the computer currently running the MySQL client (in this case the MySQL query browser) rather than the MySQL Server. The FIELDS TERMINATED BY ',' indicates that the file is comma-delimited. If the file were a tab-separated file, the statement would be FIELDS TERMINATED BY '\t'. The last portion inside parentheses tells the browser the order the fields appear in the text file, so if the zipcode were listed first in the text file, the statement would be (zipcode, name, address). Also, note the use of a forward-slash in the file location -- this is because MySQL designers use proper UNIX file locations rather than the heretic DOS-style format with a back-slash.
Now that the data is loaded, lets confirm that it is there and properly formatted in the database. Execute the following statement:
This will return all rows from the Tutorial table to the results pane of the query browser. You should see all four rows and all three columns of our original text file, now neatly organized in our database.
In the next post, I'll show you how to do more sophisticated SELECT statements. Please comment if anything is unclear and I'll do my best to clarify.
Over the course of this tutorial, I'll illustrate how to use MySQL to load, store, access, and sort SNP statistics computed by PLINK. To begin, you will need to download and install MySQL on your local machine, and you'll also need to install the MySQL GUI tools.
You can think of MySQL as a massive spreadsheet application like Excel - The basic unit within the database is a table, consisting of rows (sometimes called tuples) and columns (generally called fields). A collection of tables is called a schema (the plural form is schemata) - schemata are typically used for organizational purposes.
After installing MySQL Server and the MySQL GUI Tools, start the MySQL Query Browser. The Query Browser will prompt you for connection information - this information will depend on where you installed the MySQL Server application. Assuming that you installed the Server on your current computer, you would enter "localhost" as the host. Also, when installing MySQL Server, you were required to enter a password for the "root" account (which is the primary administrative account). Enter "root" as the username and the password you specified during setup.
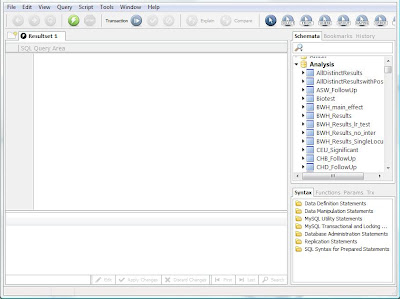
Now you should see the MySQL Query Browser, which has three main panes: the SQL query area, the schemata list, and the results pane. They schemata list is useful for browsing what the database contains, and allows you to see the field names and tables for each schema. The query editor allows you to enter and execute an SQL statement, and the results of that statement are returned in the results pane, which is essentially a read-only spreadsheet.
Lets start by creating a new schema to hold the tables we will make in this tutorial. In the Query Browser, type:
CREATE DATABASE Sandbox;Notice the semi-colon at the end of the statement - this tells the SQL engine that it has reached the end of an SQL statement. You can execute this statement two ways: 1. Hit Control-Enter, or 2. Click the button with the green lightning bolt. This statement just created a new schema in our database called Sandbox. You can see the new schema in the schemata browser if you right-click inside the schemata tab and choose refresh from the menu. The active schema in the MySQL Query Browser is always shown as bold. You can select a new active schema simply by double-clicking it in the schema browser.
Now let's create a table in the Sandbox schema. If you haven't already, double-click the Sandbox schema in the schemata browser to make it the active schema, then execute the following statement:
CREATE TABLE Tutorial (name varchar(30), address varchar(150), zipcode int);
This statement created a table called Tutorial in the Sandbox schema that has 3 fields: a character-based field with a max of 30 characters called "name", a character-based field with a max of 150 characters called "address", and an integer-based field called "zipcode".
Now let's load some data into this table. Most genetic analysis applications will output a text file with analysis results -- with this in mind, we'll create a text file containing several names, addresses, and zip codes to load into our new table:
Phillip J. Fry,Robot Arms Apartments #455,65774Copy and paste this text into a text file called "futurama_addresses.txt". Now execute the following statement:
Zapp Brannigan,Starship Nimbus Captain's Quarters,45542
Doctor Zoidberg,335 Medical Corporation Way,72552
Hubert J. Farnsworth,Planet Express Delivery Company,88754
LOAD DATA LOCAL INFILE "C:/futurama_addresses.txt" INTO TABLE Tutorial FIELDS TERMINATED BY ',' (name, address, zipcode);
The keyword LOCAL tells the MySQL browser to use files on the computer currently running the MySQL client (in this case the MySQL query browser) rather than the MySQL Server. The FIELDS TERMINATED BY ',' indicates that the file is comma-delimited. If the file were a tab-separated file, the statement would be FIELDS TERMINATED BY '\t'. The last portion inside parentheses tells the browser the order the fields appear in the text file, so if the zipcode were listed first in the text file, the statement would be (zipcode, name, address). Also, note the use of a forward-slash in the file location -- this is because MySQL designers use proper UNIX file locations rather than the heretic DOS-style format with a back-slash.
Now that the data is loaded, lets confirm that it is there and properly formatted in the database. Execute the following statement:
SELECT * FROM Tutorial;
This will return all rows from the Tutorial table to the results pane of the query browser. You should see all four rows and all three columns of our original text file, now neatly organized in our database.
In the next post, I'll show you how to do more sophisticated SELECT statements. Please comment if anything is unclear and I'll do my best to clarify.
Tags:
GWAS,
Productivity,
SQL
Wednesday, February 10, 2010
LocusZoom: Plot regional association results from GWAS
Update Friday, May 14, 2010: See this newer post on LocusZoom.
If you caught Cristen Willer's seminar here a few weeks ago you saw several beautiful figures in the style of a manhattan plot, but zoomed in around a region of interest, with several other useful information overlays.
Click to take a look at this plot below, showing the APOE region for an Alzheimer's Disease GWAS:
It's a simple plot of the -log10(p-values) for SNPs in a given region, but it also shows:
1. LD information (based on HapMap) shown by color-coded points (not much LD here).
2. Recombination rates (the blue line running through the plot). Peaks are hotspots.
3. Spatial orientation of the SNPs you plotted (running across the top)
3. Genes! The overlay along the bottom shows UCSC genes in the region.
You can very easily take a PLINK output file (or any other format) and make an image like this for your data for any SNP, gene, or region of interest using a tool Cristen and others at Michigan developed called LocusZoom. LocusZoom is written in R with a Python wrapper that works from an easy to use web interface.
All the program needs is a list of SNP names and their associated P-values. If you're using PLINK, your *.assoc or *.qassoc files have this information, but first you'll have to run a quick command to format them. Run this command I discussed in a previous post to convert your PLINK output into a comma delimited CSV file (PLINK's default is irregular whitespace delimited):
Next, you'll want to compress this file so that it doesn't take forever to upload.
Now, upload your new file (plink.assoc.csv.gz) on the LocusZoom website. Tell it that your p-value column is named "P" and your marker column is named "SNP" (or whatever they're called if you're not using PLINK). Change the delimiter type to "comma", then put in a region of interest. I chose APOE, but you could also use a SNP name (include the "rs" before the number). Now hit "Plot your Data," and it should take about a minute.
There are some other options below, but I've had bad luck using any of them. For instance, I can never get it to output a PNG properly - only PDF works the last time I tried it. I also could not successfully make a plot if I turn off the recombination rate overlay. I know this is a very early version, but hopefully they'll clean up some of the code and document some of its features very soon. I could see this being a very useful tool, especially once it's available for download for local use. (Update: some of these bugs have been fixed. See this newer post on LocusZoom).
LocusZoom: Plot regional association results from GWAS
If you caught Cristen Willer's seminar here a few weeks ago you saw several beautiful figures in the style of a manhattan plot, but zoomed in around a region of interest, with several other useful information overlays.
Click to take a look at this plot below, showing the APOE region for an Alzheimer's Disease GWAS:
It's a simple plot of the -log10(p-values) for SNPs in a given region, but it also shows:
1. LD information (based on HapMap) shown by color-coded points (not much LD here).
2. Recombination rates (the blue line running through the plot). Peaks are hotspots.
3. Spatial orientation of the SNPs you plotted (running across the top)
3. Genes! The overlay along the bottom shows UCSC genes in the region.
You can very easily take a PLINK output file (or any other format) and make an image like this for your data for any SNP, gene, or region of interest using a tool Cristen and others at Michigan developed called LocusZoom. LocusZoom is written in R with a Python wrapper that works from an easy to use web interface.
All the program needs is a list of SNP names and their associated P-values. If you're using PLINK, your *.assoc or *.qassoc files have this information, but first you'll have to run a quick command to format them. Run this command I discussed in a previous post to convert your PLINK output into a comma delimited CSV file (PLINK's default is irregular whitespace delimited):
cat plink.assoc | sed -r 's/^\s+//g' | sed -r 's/\s+/,/g' > plink.assoc.csv
Next, you'll want to compress this file so that it doesn't take forever to upload.
gzip plink.assoc.csv
Now, upload your new file (plink.assoc.csv.gz) on the LocusZoom website. Tell it that your p-value column is named "P" and your marker column is named "SNP" (or whatever they're called if you're not using PLINK). Change the delimiter type to "comma", then put in a region of interest. I chose APOE, but you could also use a SNP name (include the "rs" before the number). Now hit "Plot your Data," and it should take about a minute.
There are some other options below, but I've had bad luck using any of them. For instance, I can never get it to output a PNG properly - only PDF works the last time I tried it. I also could not successfully make a plot if I turn off the recombination rate overlay. I know this is a very early version, but hopefully they'll clean up some of the code and document some of its features very soon. I could see this being a very useful tool, especially once it's available for download for local use. (Update: some of these bugs have been fixed. See this newer post on LocusZoom).
LocusZoom: Plot regional association results from GWAS
Tags:
GWAS,
PLINK,
R,
Visualization,
Web Apps
Tuesday, February 9, 2010
Papers from Feb 8 2010 Journal Club
Here are the papers we talked about in yesterday's Journal Club:
PLoS Biol. 2010 Jan 26;8(1):e1000294.
Rare variants create synthetic genome-wide associations. See my previous coverage of this paper and the comments.
Dickson SP, Wang K, Krantz I, Hakonarson H, Goldstein DB.
PLoS Genet. 2010 Jan;6(1):e1000798. Epub 2010 Jan 8.
Modeling of environmental effects in genome-wide association studies identifies SLC2A2 and HP as novel loci influencing serum cholesterol levels.
Igl W, Johansson A, Wilson JF, Wild SH, Polasek O, Hayward C, Vitart V, Hastie N, Rudan P, Gnewuch C, Schmitz G, Meitinger T, Pramstaller PP, Hicks AA, Oostra BA, van Duijn CM, Rudan I, Wright A, Campbell H, Gyllensten U; EUROSPAN Consortium.
Science. 2010 Jan 7. [Epub ahead of print]
A Composite of Multiple Signals Distinguishes Causal Variants in Regions of Positive Selection.
Grossman SR, Shylakhter I, Karlsson EK, Byrne EH, Morales S, Frieden G, Hostetter E, Angelino E, Garber M, Zuk O, Lander ES, Schaffner SF, Sabeti PC.
PLoS Biol. 2010 Jan 26;8(1):e1000294.
Rare variants create synthetic genome-wide associations. See my previous coverage of this paper and the comments.
Dickson SP, Wang K, Krantz I, Hakonarson H, Goldstein DB.
PLoS Genet. 2010 Jan;6(1):e1000798. Epub 2010 Jan 8.
Modeling of environmental effects in genome-wide association studies identifies SLC2A2 and HP as novel loci influencing serum cholesterol levels.
Igl W, Johansson A, Wilson JF, Wild SH, Polasek O, Hayward C, Vitart V, Hastie N, Rudan P, Gnewuch C, Schmitz G, Meitinger T, Pramstaller PP, Hicks AA, Oostra BA, van Duijn CM, Rudan I, Wright A, Campbell H, Gyllensten U; EUROSPAN Consortium.
Science. 2010 Jan 7. [Epub ahead of print]
A Composite of Multiple Signals Distinguishes Causal Variants in Regions of Positive Selection.
Grossman SR, Shylakhter I, Karlsson EK, Byrne EH, Morales S, Frieden G, Hostetter E, Angelino E, Garber M, Zuk O, Lander ES, Schaffner SF, Sabeti PC.
Tags:
Journal club,
Recommended Reading
Monday, February 8, 2010
Regression Modeling Strategies Course by Frank Harrell
Frank Harrell is teaching his 3-session short course on regression modeling strategies using R here at Vanderbilt next month. Frank is a professor and chair of the Vanderbilt Biostatistics Department, and the author of several massively popular R libraries, including Design, rms, and the indispensable Hmisc. He has also written a book, covering many topics related to regression modeling (Amazon, $98). You can find more information about the course and registration at this link.
The course consists of three half days:
Wednesday, March 31 (8:00AM-12:00PM)
Thursday, April 1 (8:00AM-12:00PM)
Friday, April 2 (8:00AM-4:00PM)
Registration Fees:
VU and MMC Students and Post-docs $50
VU and MMC Faculty and Staff $200
Other Students $200
Other Members of Non-Profit Institutions $400
Members of For-Profit Institutions $600
No charge to Department of Biostatistics faculty/staff
Regression Modeling Strategies - 2010 Short Course
The course consists of three half days:
Wednesday, March 31 (8:00AM-12:00PM)
Thursday, April 1 (8:00AM-12:00PM)
Friday, April 2 (8:00AM-4:00PM)
Registration Fees:
VU and MMC Students and Post-docs $50
VU and MMC Faculty and Staff $200
Other Students $200
Other Members of Non-Profit Institutions $400
Members of For-Profit Institutions $600
No charge to Department of Biostatistics faculty/staff
Regression Modeling Strategies - 2010 Short Course
Tags:
Announcements,
R,
Statistics
Friday, February 5, 2010
Computational Genomics Journal Club, Monday Feb 8
We're restarting the PCG Journal Club again Monday February 8 at 4pm in the CHGR conference room. Most of you who usually attend are familiar with the format, but if not, bring any papers you've read recently and give a brief (i.e. 2 minute) overview of the paper and why you thought it was interesting. No slides allowed. We're also going to discuss the previously mentioned Rare Variants Create Synthetic Associations paper recently published in PLoS Biology, which has generated a bit of controversy lately. Oh yeah, and we'll have beer and snacks. In case you miss it, Julia will be posting links to all the papers we discuss under the Journal Club tag. You can also view any of the papers from previous journal clubs under that link.
Tags:
Announcements,
Journal club
Research Symposium at HudsonAlpha Institute for Biotechnology
If you were here for any of the talks Rick Myers has given here at Vanderbilt over the last few years you'll remember all the interesting biomedical research going on at his company, HudsonAlpha. Their spring symposium is March 30, 8am-6pm, at the HudsonAlpha institute in Huntsville, AL. It's FREE, and poster sessions are open to all students and postdocs. Participating institutions include HudsonAlpha, UAB, Auburn University, Emory University, The University of Alabama, and of course Vanderbilt University. See the link below for registration.
HudsonAlpha Institute for Biotechnology: Spring Symposium
HudsonAlpha Institute for Biotechnology: Spring Symposium
Tags:
Announcements
Thursday, February 4, 2010
Qualifying exam study guide phase I
Vanderbilt 2nd year grad students: Here is the study guide I made for studying for my general knowledge phase I qualifying exam. I'd recommend making your own, but this may help you with a place to start. You can download it at the link below.
Update January 25, 2013: I've uploaded the link to Figshare for a more permanent home.
http://dx.doi.org/10.6084/m9.figshare.154339
Update January 25, 2013: I've uploaded the link to Figshare for a more permanent home.
http://dx.doi.org/10.6084/m9.figshare.154339
Monday, February 1, 2010
GRAIL: Gene Relationships Across Implicated Loci
If you caught Soumya Raychaudhuri's seminar last week you heard a lot about the tool he developed at the broad called GRAIL - Gene Relationships Across Implicated Loci. You've got GWAS results and now you want to prioritize SNPs to follow up in replication or functional studies. Of course you're going to take your stellar hits at p<10e-8, but what about that fuzzy region between 10e-4 and 10e-8? Here's where a tool like GRAIL may come in handy.
In essence, you feed GRAIL a list of SNPs and it maps these SNPs to gene regions using LD. It then uses a simple text-mining algorithm to ascertain the degree of connectivity among the associated genes by looking at the similarity of vectors of words pulled from PubMed abstracts which mention your gene of interest. In their most recent paper they took a list of 370 GWAS hits, and narrowed this down to a list of 22 candidate SNPs to follow up. And it turns out these SNPs replicated in an independent set at a much higher frequency than random SNPs from the subset of 370. In his talk, Soumya offered convincing evidence that using the results from GRAIL you have a much better shot at replicating associations than if you just looked at the p-value rankings alone. After the talk he did mention that this approach has had mixed success depending on the phenotype. Here's the original GRAIL paper, and the OpenHelix blog has a nice 5-minute video screencast demonstration of GRAIL where they take a few SNPs from the GWAS catalog and run them through GRAIL.
GRAIL is a free web application (beta) available at the Broad's website below.
GRAIL: Gene Relationships Across Implicated Loci
Tags:
Bioinformatics,
GWAS,
Pathways,
Software,
Web Apps
Subscribe to:
Posts (Atom)